

Satellite images, such as those in Google Street View, are taking on new purpose in the hands of Chaofeng Wang, Ph.D., a recent AI hire in UF’s M.E. Rinker, Sr. School of Construction Management.
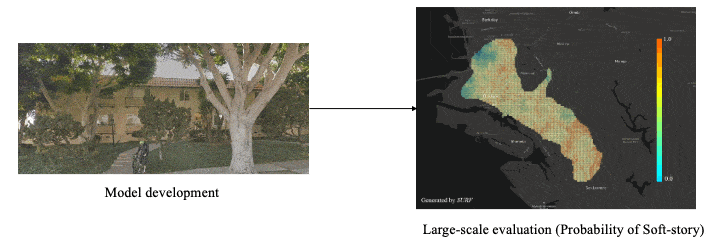
With the help of HiPerGator, Wang is training AI programs to analyze Street View images to automate how urban buildings are evaluated for structural soundness. His project aims to help governments and other entities mitigate the damage caused by natural disasters and help decision makers conduct post-disaster assessments more quickly and efficiently.
The on-site process of evaluating a city after disaster strikes can take months. Wang’s method reduces that time to a few hours.
“Without NVIDIA GPUs [graphics processing units], we wouldn’t have been able to do this,” Wang said. “They significantly accelerate the process, ensuring timely results.”
Wang’s AI model is trained to assign scores to buildings based on federal standards, with calibrations for structure type, wall type and building age. Wang also sourced building styles from around the world to improve the model’s accuracy.
WHY IT MATTERS
New AI tool uses Google Street View for faster, safer disaster relief
The AI model’s results are compiled in a database linked to a web portal, which displays the information in map formats. The information can be used for a variety of purposes, Wang notes, including disaster assessment, insurance evaluation and, with more tweaks, disaster preparedness, structural rehabilitation and urban planning.
Local governments in Mexico are already testing the project, and countries in Africa, Asia and South America have expressed interest. In its current iteration, the model can achieve more than 85 percent accuracy in its assessment scores, per federal standards.